PhD Proposal: Harley Edwards
FORMAT: VIRTUAL PRESENTATION
Location
Online
PhD Proposal: Harley Edwards – Online Event
Date & Time
January 15, 2021, 11:00 am – 12:00 pm
Description
Harley Edwards, PhD Student
Advisor: Dr. Mark Marten
Title:
Using symbolic regression to infer phosphoproteomic interactions in A. nidulansAs scientists in parallel add to their own island of knowledge, engineers build the bridges between them, with roads that all lead back to practical application. This work is a bridge between machine learning and phosphoproteomics, with practical application in reconstructing eukaryotic signal transduction pathways. Phosphoproteomic research spans disciplines from fungal biology to human medicine. Regardless of the industry, or the organism, common to all phosphoproteomic researchers is some version of phosphopeptide enriched mass spectrometry, which has long been used to probe the phosphorylation state of a cell line under different conditions. Modern methods create quantitative signals, peaks, belonging to lists of thousands of phosphosites. By comparing these lists under two different conditions, scientists deduce information based on what changes, or what stays the same. Owed to the success of this kind of experiment, the increased availability/performance of mass spectrometers, and the advancements in automation, high dimensional phosphoproteomic data is created in a high throughput fashion. Phosphoproteomic data analysis uses statistical and bioinformatic approaches, similarly to other omics fields, like cluster profiling and database curation, to sort, group, and manage the data about these thousands of phosphosites. While bioinformatic approaches help identify patterns and manage data, there is a need for unbiased reconstruction of phosphoproteomic signaling networks based on empirical data and without a priori database reference. The main objective is to benchmark current methods and apply them to infer differential equation models from experimental data. Our central hypothesis is that SRvGP will infer descriptive correlations between phosphorylation sites from phosphoproteomic mass spectrometry data. Before using this algorithm on datasets where the underlying model is unknown, we need to validate our current methods on datasets from biologically and experimentally relevant models which we know. To validate the performance of the algorithm for use when the solution is unknown, objective criteria are needed to establish algorithm convergence. Similarly, objective rejection criteria are needed for determining when a model is to be rejected from our ensemble. With established algorithm performance metrics and model rejection criteria, the next step is to gauge the success of the algorithm when used to infer biologically relevant models of increasing size and noise, from data generated in silico. Using previously mentioned success criteria, and within the bounds of what was found to work in silico, SRvGP will be used to infer differential equations governing phosphosite occupancy, in terms of other phosphosites, and based on real phosphopeptide enriched mass spectrometry data describing in vivo biological samples. In summary, the goal is to establish benchmarks to objectively determine algorithm/model success, validate the algorithm on biological models from data generated in silico, and then describe the performance of this algorithm when applied to biological models from data generated in vivo.
Agenda:
- 10:45 am: Meeting room will open
- 11:00 AM: 1 hr presentation will be open to the public with Q&A.
- Followed by a closed session with the committee and PhD Student.
Meeting link:
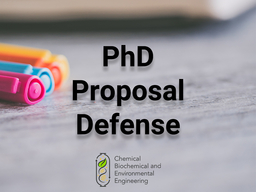